Gartner: 10 changes coming to data analytics
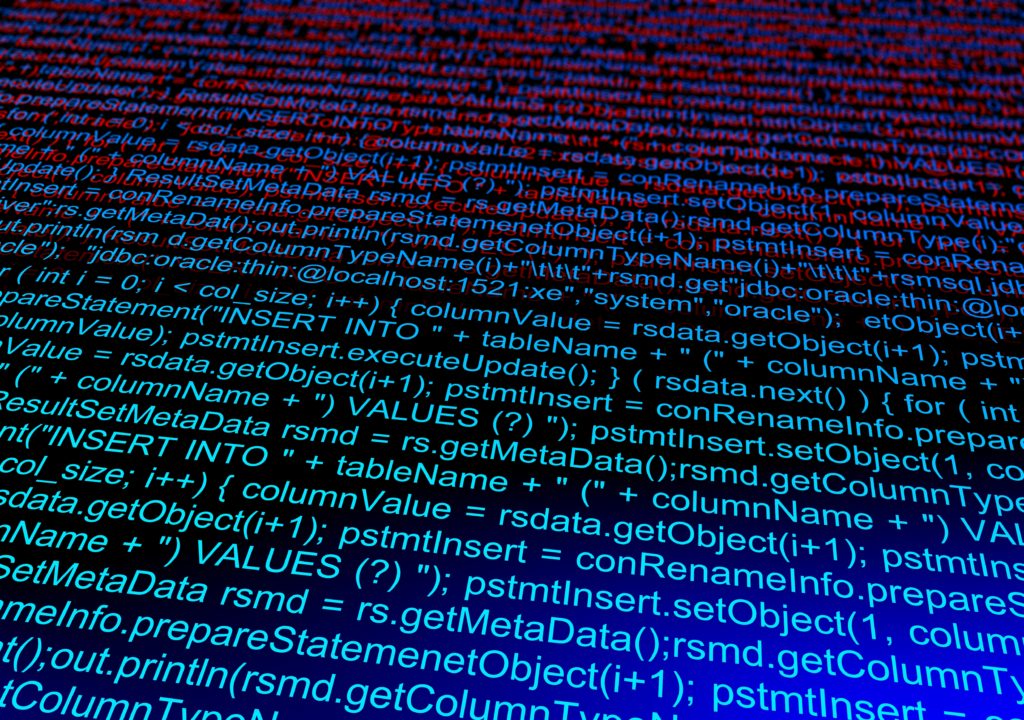
Original article available here.
Businesses that trust AI to operate will leverage different kinds of data input and infuse automation into how they extract insights.
The year began with an ambitious data mandate for organizations: leverage data analytics and AI techniques to keep up with the competition and increase efficiency.
Pressed by the challenges of a redrawn business landscape, leaders searched for guidance in their data and analytics toolkit. In the pivot to distributed work, AI helped field rising help desk requests from a mobile workforce. Data analytics informed leaders in near-real time how consumption patterns shifted, helping manage supply chain constraints.
Radical change and uncertainty now challenges organizations to forge new paths within their data and analytics strategies, said Rita Sallam, distinguished VP analyst at Gartner speaking at a Gartner IT Symposium/Xpo Americas session last week.
“It’s not just to make it to the other side, but to thrive when we get there,” Sallam said.
Organizations emerging from the initial reactive phase will look to their data analytics initiatives to enable a smarter company, one that automates insight generation and finds ways to monetize its data inventory.
Diverse types of data — such as audio and video — will come under the magnifying glass to equip companies with richer insight into their operations.
Here are 10 trends set to shape businesses’ data strategies:
Smarter, faster, more responsible AI
Initially groundbreaking and available to a select few, access to AI techniques has expanded across company departments. More enterprises wll operationalize their AI initiatives by 2024, according to Gartner.
But “if users don’t trust data, if they can’t understand how a model works, the more complex it gets, the more opaque it typically gets, the less likely they are to trust that model and use it,” said Sallam. Organizations need to arrive at AI strategies that augment employees in their daily work and decisions.
AI that’s smarter, faster and more responsible powers the applications technology that can help cities manage traffic flow, assist doctors in diagnosing illnesses or applying algorithms in near-real time to manage time-sensitive tasks in the financial market.
Still, challenges lie ahead. Data sets may no longer be accurate at predicting outcomes amid disruption induced by COVID-19.
Decline of the dashboard
Dashboards are a ubiquitous tool in platforms that promise AI-driven insights. A quickly adjustable tool to let analysts and middle management arrive at conclusions from available data sets.
“The predefined dashboard with predefined KPIs, predefined relationships, is likely to be displaced,” Sallam said.
In its stead, platforms will combine techniques such as augmented analytics, natural language processing and anomaly detection to keep users from performing tasks associated with analysis.
But Gartner projects that by 2025, data stories will be the most widespread way of consuming analytics, with 75% of those stories automatically generated through augmented analytics techniques.
Decision intelligence
By 2023, Gartner projects more than one-third of large organizations will have analysts practicing decision intelligence, including decision modeling.
Decision intelligence includes a range of decision-making techniques such as rules-based approaches to AI and machine learning in order to fine-tune decision-making, Sallam said.
Though current adoption remains low, these techniques are used by financial services companies to assist in deciding mortgage applications.
Enterprise adoption will increase, with business leaders lured by the reduction in time and effort it delivers to the design, development and deployment of complicated business logic.
X analytics
The types of data points that companies use to make decisions will expand, with organizations reaching into video, audio, olfactory, vibration, natural language, sentiment or emotion data to derive actionable insights.
In the term “X analytics,” X works as a variable that can be replaced with any type of content.
“Over the past 10 years, with the rise of big data, we’ve done a great job at storing and managing content, or X data,” said Sallam. “What we haven’t done is a great job at using that pervasively across the organization.”
By 2025, AI for video, audio, vibration, text, emotion and other content analytics will trigger significant innovations and transformations at more than three-quarters of Fortune 500 companies, according to Gartner.
Some examples of these data points include analyzing video and audio data for supply chain optimization, predictive maintenance traffic management or inventory optimization.
Metadata is the new black
Richness in data leads to challenges in its management. The ability to analyze data across silos through machine learning is at the center of an architectural concept called data fabric, where business use continuous analytics over existing, discoverable and inferred metadata assets.
By 2023, organizations utilizing active metadata, machine learning and data fabrics to dynamically connect and automate data management processes will reduce their time to data delivery, and impact on value by 30%, Gartner expects.
The strategy will let businesses rapidly plug in new types of data regardless of where it sits and how it is formatted, in order to support new types of analytics, said Sallam.
Cloud is a given
Prior to the pandemic, the trend was already clear: there was a significant acceleration of organizations moving their data management, analytics, machine learning and AI workloads to the cloud.
Expect this to continue and accelerate, with cloud-based AI increasing fivefold by 2023, Sallam said. This spike would make AI one of the top workloads in the cloud.
“Public cloud services make it possible for us to do lots of innovation rapidly,” said Sallam. It’s led many leaders to move to the cloud in search of a more agile AI strategy that accelerates change.
Data and analytics worlds collide
Convergence lies ahead for analytics and business intelligence platforms, with many vendors adding on-board data management capabilities, from data preparation to data cataloging and data profiling.
Platforms will aim to “enable end-to-end flows within single platforms,” and making these tools accessible “to less skilled users,” said Sallam.
By 2022, Gartner expects 40% of machine learning model development scoring will be done in products that don’t have machine learning as their primary goal.
Data marketplaces/exchanges
If data has long been “the new oil,” then organizations searching for additional revenue streams will look to break out the drills and monetize what they own.
The robustness of data-sharing capabilities will rise in importance. In the next two years, more than one-third of large organizations will be either selling or buying data via online marketplaces, Gartner projects.
The prediction marks an increase from current corporate presence in data marketplaces. Just one-quarter of companies currently buy or sell data through such marketplaces as of 2020.
Practical blockchain (for data and analytics)
Blockchain shouldn’t become a science project within the company’s tech stack. An ideal blockchain deployment should aim to find an adequate fit between the use case and the technology’s capabilities.
Successful initiatives let business outcomes drive deployment, with payment settlements and providence asset tracking as examples, said Sallam.
By 2023, Gartner projects companies using blockchain smart contracts will increase overall data quality by 50%, but reduce data availability by 30%, “conversely creating positive data and analytics ROI.”
Relationships form the foundation of data and analytics value
Organizations need to efficiently relate multiple data sets, such as people, places, things or locations.
“And that often impacts performance, Sallam said. “The more tables we join together, the more the more complex data, we see performance degrade.”
But graph modeling techniques allow brands to establish relations between products people are more likely to buy alongside another item. Graph analytics will facilitate rapid contextualization for decision making in 30% of organizations worldwide by 2023.